CRC算法原理与实现系列文章:
CRC算法原理与实现02——数学原理与模2运算 – 徐晓康的博客
CRC算法原理与实现03——参数说明、计算步骤与应用场景 – 徐晓康的博客
CRC算法原理与实现04——软硬件实现方法简介 – 徐晓康的博客
CRC算法原理与实现05——并行计算公式推导 – 徐晓康的博客
CRC算法原理与实现06——自编Python计算任意CRC – 徐晓康的博客
CRC算法原理与实现07——Verilog单步计算任意CRC – 徐晓康的博客
CRC算法原理与实现08——Verilog多步计算任意CRC – 徐晓康的博客
CRC算法原理与实现09——C语言计算任意CRC – 徐晓康的博客
前言
本文是CRC并行计算的理论基础。为了理解CRC并行计算的原理,我看了很多论文,这套方法在一些论文中应该已经讲过了,但遗憾的是,很少有文章能把它讲清楚。本文从最基础的数学原理出发,力求详细且不跳步,有点线性代数基础的同学应该能顺利理解。
并行算法是从基础的移位寄存器法中总结出来的,下面详细推导出并行计算的公式。
一. CRC并行计算公式推导
以串行左移反馈移位寄存器
为参考,考虑用数学公式按左移反馈的步骤,一步步推导出并行计算的公式。
设宽度为n的CRC寄存器的初始值为C,用行向量表示,有:
生成多项式用G表示,有:
设输入数据的位宽为k,用行向量D表示,有:
设CRC寄存器左移移位并反馈后的状态为,有:
根据左移反馈的运算机制,当原寄存器的最高位为1时,CRC左移一位,并于生成多项式G进行异或;当原寄存器最高位为0时,CRC左移一位,不异或,由此可得到如下递推关系:
这里的所有符号变量的值都是二进制的,不是0就是1,注意为原寄存器的最高位的值。
两个数的异或运算,等价于两个数加法后模2
,所以上式可改写为:
根据上述表达式,可以构建与C之间的状态转移矩阵表达式,称为
单步状态转移公式
,有:
其中,T为状态转移矩阵,它第一行为生成多项式G的系数
,左下为n-1×n-1的单位矩阵
,右下全0,有:
为输入向量的扩展形式,为n个元素的行向量,最后一个元素为
,即输入数据的最高位,其余位全0,有:
我们来验证一下这个表达式是否正确,有:
根据矩阵乘法运算的规则,有:
所以,这里证明了单步状态转移公式
的正确性。
根据这个公式进一步推导,我们就能得到多步状态转移公式
,也就是并行计算公式
了。
现在,考虑下一步的状态
,根据单步状态转移公式,有:
其中,,它表示原始数据的次高位现在移入寄存器了。
把展开,有:
这个表达式就有点复杂了,考虑mod2运算的性质:
矩阵A与B的乘积模2等于A模2乘以B模2
。另一个性质:
矩阵A与B的和模2等于A模2和B模2的和再模2
。
因为T和每个元素为0或1,所以,
所以,可以写为:
下面计算一下,,有:
所以,可以认为对
的作用是:使
左移一位,低位补0。
考虑,有:
令,
令,这里
可以理解为原始数据D左移进入一个初始值全0的寄存器,左移了两位,有:
那么的下一个状态
呢,容易推导出:
其中,。
到这里规律就显而易见了,因为’,” 和 ”’表示的步骤有限,令:
状态转换公式可写为:
注意:
-
为CRC寄存器的初始值,对于从最初开始计算CRC来说,
它总是0
。只有分多步计算CRC时,前面已经算过至少一次了,C(0)作为后续步骤的CRC寄存器初始值。 -
这里隐含了数学上对矩阵零次方的定义,即一个n×n的矩阵的零次方 = 单位矩阵,即对角线元素为1,其余位全0的矩阵。
从上述公式我们可以看出,i为移位的步数,状态转移矩阵T由生成多项式决定,D(n)由输入数据和计算步数决定,所以,这里就得到了移位寄存器移动i位后的状态与生成多项式和输入数据的关系。
此公式是并行CRC计算的理论基础
。
二. CRC并行计算公式验证
2.1 CRC-8
-
多项式: 0x07
(0000_0111
) -
初始异或值: 0x00
-
输入反转:无 -
输出反转:无 -
最终异或值: 0x00
输入数据为0x12,注意在进行CRC计算时,输入数据需要先补0再异或,所以实际参与运算的输入数据为0x1200,有:
CRC寄存器初始值为0,有:
以C(8)为初始值,有最终的CRC寄存器值:
其中,为输入数据的第9~16位,实际是补的0。有:
这个我们写个Python程序算一下:
import numpy as np
# 定义矩阵 D(8)
D = np.array([[0, 0, 0, 1, 0, 0, 1, 0]])
# 定义矩阵 T
T = np.array([
[0, 0, 0, 0, 0, 1, 1, 1],
[1, 0, 0, 0, 0, 0, 0, 0],
[0, 1, 0, 0, 0, 0, 0, 0],
[0, 0, 1, 0, 0, 0, 0, 0],
[0, 0, 0, 1, 0, 0, 0, 0],
[0, 0, 0, 0, 1, 0, 0, 0],
[0, 0, 0, 0, 0, 1, 0, 0],
[0, 0, 0, 0, 0, 0, 1, 0]
])
# 计算 T^8
T_power_8 = np.linalg.matrix_power(T, 8)
# 计算 C(16) = D(8) * T^8
C = np.dot(D, T_power_8) % 2
# 输出结果
print("T^8:")
print(T_power_8)
print("\nC(16):")
print(C)
运行结果:
T^8:
[[1 0 0 0 1 2 2 1]
[1 1 0 0 0 1 1 1]
[1 1 1 0 0 0 0 0]
[0 1 1 1 0 0 0 0]
[0 0 1 1 1 0 0 0]
[0 0 0 1 1 1 0 0]
[0 0 0 0 1 1 1 0]
[0 0 0 0 0 1 1 1]]
C(16):
[[0 1 1 1 1 1 1 0]]
对照CRC计算网站上计算的结果:两者一致。
2.2 CRC-16-CCITT-FALSE
-
多项式: 0x1021
(0001_0000_0010_0001
) -
初始异或值: 0xFFFF
-
输入反转:无 -
输出反转:无 -
最终异或值: 0x0000
输入数据0x5678,先补0变为0x56780000,再与初始异或值异或,为0xA9870000,推导一下输出表达式:
对应Python程序:
import numpy as np
# 定义矩阵 D(16) 0xA987
D16 = np.array([[1, 0, 1, 0, 1, 0, 0, 1, 1, 0, 0, 0, 0, 1, 1, 1]])
# 定义矩阵 T, G=0x1021
T = np.array([
[0, 0, 0, 1, 0, 0, 0, 0, 0, 0, 1, 0, 0, 0, 0, 1],
[1, 0, 0, 0, 0, 0, 0, 0, 0, 0, 0, 0, 0, 0, 0, 0],
[0, 1, 0, 0, 0, 0, 0, 0, 0, 0, 0, 0, 0, 0, 0, 0],
[0, 0, 1, 0, 0, 0, 0, 0, 0, 0, 0, 0, 0, 0, 0, 0],
[0, 0, 0, 1, 0, 0, 0, 0, 0, 0, 0, 0, 0, 0, 0, 0],
[0, 0, 0, 0, 1, 0, 0, 0, 0, 0, 0, 0, 0, 0, 0, 0],
[0, 0, 0, 0, 0, 1, 0, 0, 0, 0, 0, 0, 0, 0, 0, 0],
[0, 0, 0, 0, 0, 0, 1, 0, 0, 0, 0, 0, 0, 0, 0, 0],
[0, 0, 0, 0, 0, 0, 0, 1, 0, 0, 0, 0, 0, 0, 0, 0],
[0, 0, 0, 0, 0, 0, 0, 0, 1, 0, 0, 0, 0, 0, 0, 0],
[0, 0, 0, 0, 0, 0, 0, 0, 0, 1, 0, 0, 0, 0, 0, 0],
[0, 0, 0, 0, 0, 0, 0, 0, 0, 0, 1, 0, 0, 0, 0, 0],
[0, 0, 0, 0, 0, 0, 0, 0, 0, 0, 0, 1, 0, 0, 0, 0],
[0, 0, 0, 0, 0, 0, 0, 0, 0, 0, 0, 0, 1, 0, 0, 0],
[0, 0, 0, 0, 0, 0, 0, 0, 0, 0, 0, 0, 0, 1, 0, 0],
[0, 0, 0, 0, 0, 0, 0, 0, 0, 0, 0, 0, 0, 0, 1, 0]
])
# 计算 T^16
T_power_16 = np.linalg.matrix_power(T, 16)
# 计算 C(32) = D(16) * T^16
C = np.dot(D16, T_power_16)
C = C % 2
# 输出结果
print("T^16:")
print(T_power_16)
print("\nC(32):")
print(C)
运行结果:
T^16:
[[2 0 0 3 1 0 1 1 1 0 2 1 1 0 0 2]
[2 2 0 0 1 1 0 1 1 1 0 0 1 1 0 0]
[0 2 2 0 0 1 1 0 1 1 1 0 0 1 1 0]
[0 0 2 2 0 0 1 1 0 1 1 1 0 0 1 1]
[1 0 0 2 1 0 0 1 1 0 1 0 1 0 0 1]
[1 1 0 0 1 1 0 0 1 1 0 0 0 1 0 0]
[0 1 1 0 0 1 1 0 0 1 1 0 0 0 1 0]
[0 0 1 1 0 0 1 1 0 0 1 1 0 0 0 1]
[1 0 0 1 0 0 0 1 1 0 0 0 1 0 0 0]
[0 1 0 0 1 0 0 0 1 1 0 0 0 1 0 0]
[0 0 1 0 0 1 0 0 0 1 1 0 0 0 1 0]
[0 0 0 1 0 0 1 0 0 0 1 1 0 0 0 1]
[1 0 0 0 0 0 0 1 0 0 0 0 1 0 0 0]
[0 1 0 0 0 0 0 0 1 0 0 0 0 1 0 0]
[0 0 1 0 0 0 0 0 0 1 0 0 0 0 1 0]
[0 0 0 1 0 0 0 0 0 0 1 0 0 0 0 1]]
C(32):
[[0 1 0 0 0 1 1 0 1 0 0 0 1 0 0 1]]
对照CRC计算网站上计算的结果:两者一致。
三. 通用CRC并行计算公式推导
回顾一下上文推导的CRC并行公式:
此公式一次可以并行计算i位,i最大为CRC的宽度n,意思是最多一次算n位。但输入数据在补0后,位宽总是比CRC宽度要大,有没有公式可以一次性算完所有位呢?当然可以,只需要基于这个公式再推导一下:
设CRC位宽为N,用大写N替代小写n,表示对于一个确定的CRC,位宽总是固定的,有:
将视为一下步计算的CRC寄存器初始状态,有:
由此式可以推导出,的表达式:
那么超过2N个bit呢?有:
由此可以总结出规律:
这就是通用的CRC并行计算公式
,它实现了任意位宽数据的并行计算
。
四. 通用CRC并行计算公式验证
4.1 CRC-8
输入数据为0x1234567,补0后为0x123456700,一次算完:
编写Python代码:
import numpy as np
# 定义矩阵 D(8) D(9:16) D(17:24) D(25:32)
D8 = np.array([[0, 0, 0, 1, 0, 0, 1, 0]]) # 0x12
D9_16 = np.array([[0, 0, 1, 1, 0, 1, 0, 0]]) # 0x34
D17_24 = np.array([[0, 1, 0, 1, 0, 1, 1, 0]]) # 0x56
D25_32 = np.array([[0, 1, 1, 1, 0, 0, 0, 0]]) # 0x70
# 定义矩阵 T
T = np.array([
[0, 0, 0, 0, 0, 1, 1, 1],
[1, 0, 0, 0, 0, 0, 0, 0],
[0, 1, 0, 0, 0, 0, 0, 0],
[0, 0, 1, 0, 0, 0, 0, 0],
[0, 0, 0, 1, 0, 0, 0, 0],
[0, 0, 0, 0, 1, 0, 0, 0],
[0, 0, 0, 0, 0, 1, 0, 0],
[0, 0, 0, 0, 0, 0, 1, 0]
])
# 计算 T^28 T^20 T^12 T^4
T_power_28 = np.linalg.matrix_power(T, 28)
T_power_20 = np.linalg.matrix_power(T, 20)
T_power_12 = np.linalg.matrix_power(T, 12)
T_power_4 = np.linalg.matrix_power(T, 4)
# 计算 C(36) = D(8)*T^28 + D(9:16)*T^20 + D(17:24)*T^12 + D(25:32)*T^4
C = np.dot(D8, T_power_28) \
+ np.dot(D9_16, T_power_20) \
+ np.dot(D17_24, T_power_12) \
+ np.dot(D25_32, T_power_4)
C = C % 2
# 输出结果
print("\nC(36):")
print(C)
运行结果:
C(36):
[[1 1 0 0 0 0 0 0]]
对照CRC计算网站上计算的结果:两者一致。
4.2 CRC-16-CCITT-FALSE
略,相信各位同学已经理解了公式,自行验证一下吧。
如果本文对你有所帮助,欢迎点赞、转发、收藏、评论让更多人看到,赞赏支持就更好了。
如果对文章内容有疑问,请务必清楚描述问题,留言评论或私信告知我,我看到会回复。
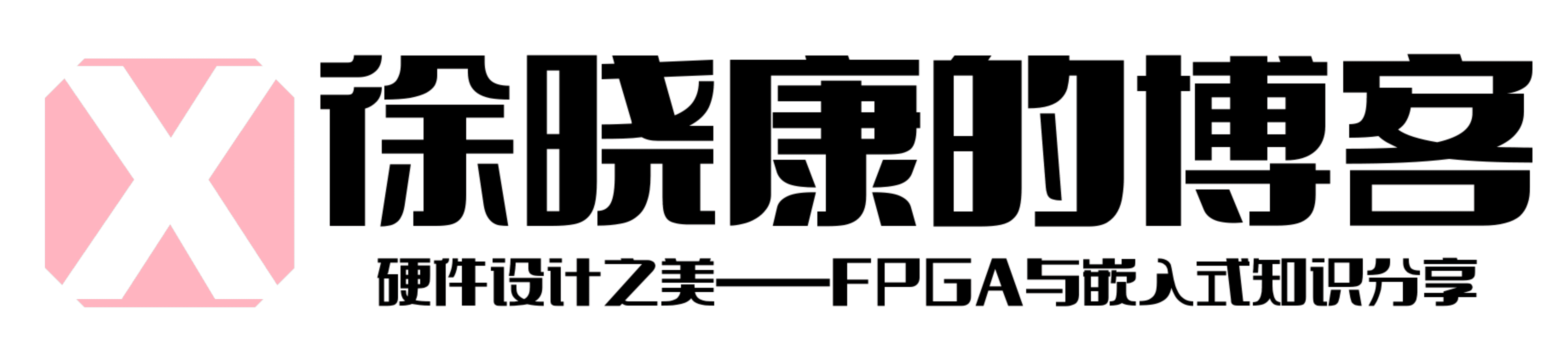
徐晓康的博客持续分享高质量硬件、FPGA与嵌入式知识,软件,工具等内容,欢迎大家关注。